The Role of Automated Defect Detection in Web Inspection Systems
Whether it’s in the realms of printing, packaging, textiles, or manufacturing, defects in materials can lead to significant losses in revenue and reputation. Web inspection systems have emerged as indispensable tools in identifying defects in continuous materials such as paper, textiles, and films. Among the most significant advancements in these systems is the integration of automated defect detection, which enhances accuracy, efficiency, and overall production quality.
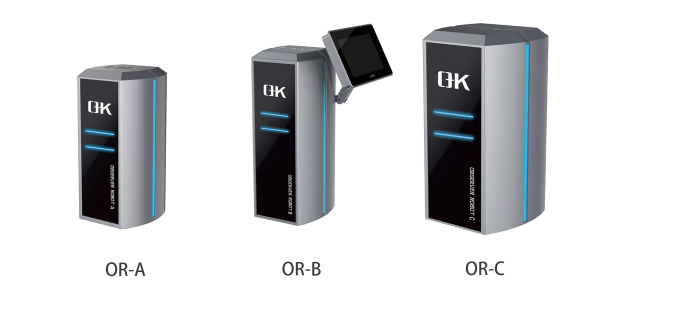
The Importance of Automated Defect Detection in Web Inspection Systems
Understanding Web Inspection Systems
Web inspection systems are specialized machines used to examine continuous materials, or “webs,” for defects during manufacturing processes. These defects can range from minor imperfections to critical flaws that render the product unusable. Traditionally, operators performed visual inspections, but this method is prone to errors due to human fatigue and subjectivity. Moreover, it is inefficient for high-speed production lines. Web inspection systems address these challenges by employing various technologies such as cameras, sensors, and image processing algorithms to detect defects accurately and swiftly.
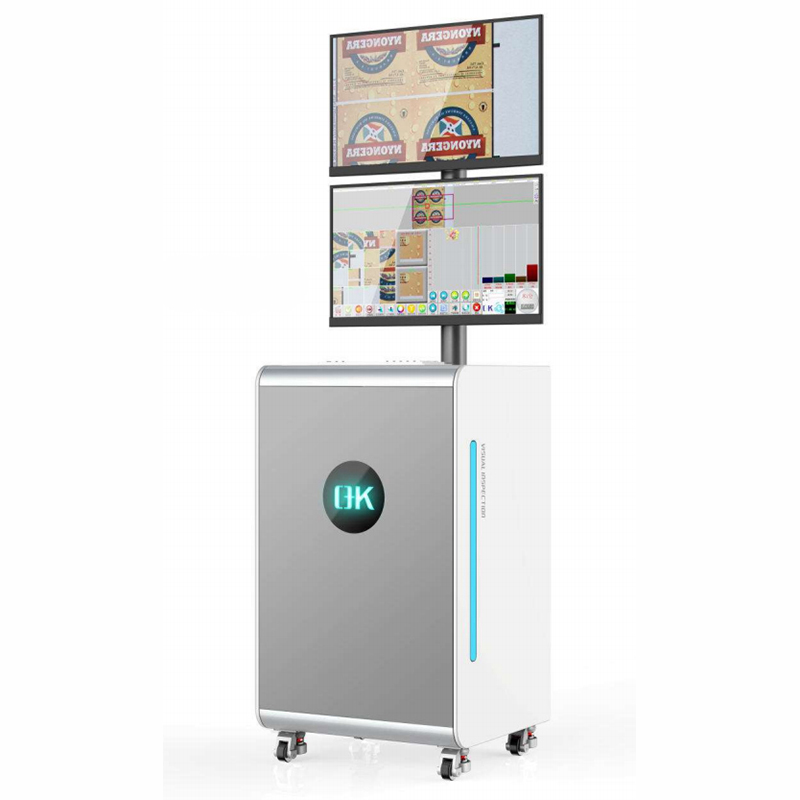
Automated Defect Detection: A Game Changer
Automated defect detection represents a significant leap forward in quality assurance within web inspection systems. By harnessing the power of artificial intelligence, machine learning, and computer vision, these systems can quickly and accurately identify defects in real-time, minimizing production downtime and waste.
Benefits of Automated Defect Detection in Web Inspection Systems
1. Improved Accuracy
Automated defect detection systems employ advanced algorithms and machine learning techniques to analyze images and identify defects with a high level of precision. This accuracy ensures that even minor defects, which may be missed by human inspectors, are detected reliably, leading to higher-quality products.
2. Enhanced Efficiency
Unlike manual inspection methods, which are time-consuming and labor-intensive, automated defect detection systems can inspect materials at high speeds without sacrificing accuracy. This enables manufacturers to maintain efficient production rates and meet tight deadlines while ensuring thorough quality control.
3. Consistency
Automated systems provide consistent performance over extended periods, eliminating the variability associated with human inspectors. This consistency ensures uniform quality across all production batches, reducing the likelihood of defects escaping detection and reaching consumers.
4. Cost Savings
By detecting defects early in the production process, automated systems help minimize waste, rework, and scrap costs. Additionally, the prevention of defective products from reaching the market reduces the potential for costly recalls, warranty claims, and damage to brand reputation.
5. Real-time Monitoring
Automated defect detection systems provide real-time feedback on the quality of materials being produced, allowing manufacturers to identify and address issues promptly. This proactive approach helps prevent defects from escalating into larger problems, minimizing production downtime and maximizing yield.
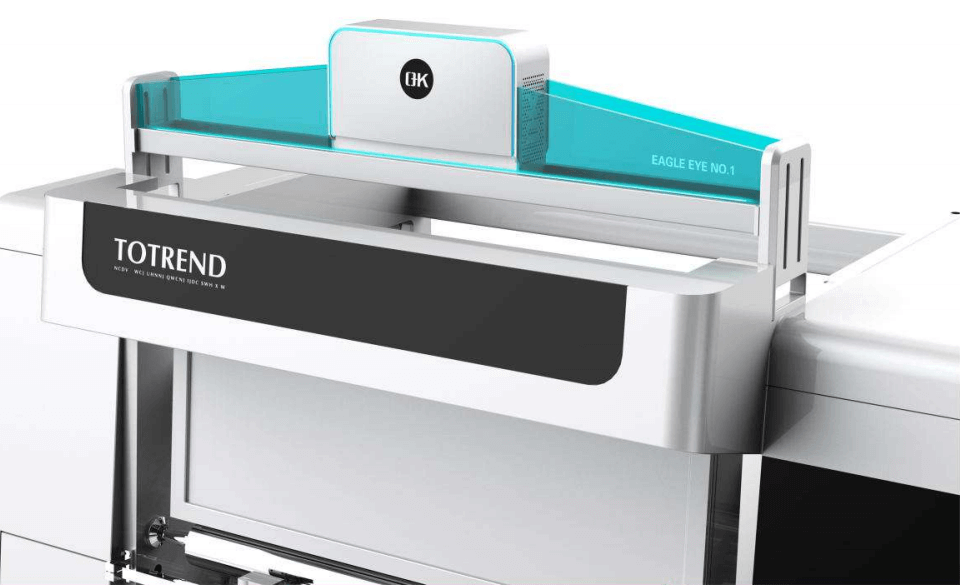
6. Data Insights
Automated defect detection systems generate valuable data insights that can be used to optimize production processes and improve overall quality. By analyzing patterns and trends in defect occurrence, manufacturers can identify areas for improvement, refine product designs, and enhance manufacturing practices.
7. Adaptability
Automated defect detection systems can be easily configured and adapted to accommodate different materials, production environments, and inspection requirements. This flexibility ensures that manufacturers can effectively detect defects across a wide range of products and applications, without the need for extensive reprogramming or reconfiguration.
Technologies Driving Automated Defect Detection in Web Inspection Systems
Automated defect detection in web inspection systems relies on a combination of cutting-edge technologies to accurately identify and analyze defects in continuous materials.
1. Machine Vision
Machine vision serves as the foundation for automated defect detection systems. It involves the use of cameras, sensors, and optics to capture high-resolution images or videos of the material being inspected. These images are then processed using specialized software algorithms to detect defects such as surface irregularities, color variations, and dimensional discrepancies.
2. Artificial Intelligence (AI) and Machine Learning
AI algorithms, particularly those based on machine learning techniques such as neural networks, play a crucial role in automated defect detection. By training on large datasets containing examples of defects and non-defective materials, these algorithms can learn to recognize patterns and anomalies indicative of defects. As they are exposed to more data, they become increasingly accurate in identifying defects, even in complex or subtle forms.
3. Deep Learning
Deep learning, a subset of machine learning, has emerged as a powerful tool for automated defect detection. Deep neural networks, comprising multiple layers of interconnected nodes, can automatically extract features from images and learn hierarchical representations of defects. This enables them to detect defects with exceptional accuracy and robustness across diverse materials and production environments.
4. Image Processing Algorithm
Image processing algorithms are essential for extracting relevant information from captured images and enhancing the visibility of defects. These algorithms may include techniques such as edge detection, thresholding, morphology, and texture analysis, which help highlight and isolate defects from background noise or variations in lighting conditions.
5. Sensor Integration
In addition to visual inspection using cameras, web inspection systems may incorporate various sensors to detect defects based on physical properties such as thickness, color, temperature, or chemical composition. These sensors provide complementary information that enhances the overall defect detection capabilities of the system.
6. Data Fusion
Data fusion techniques integrate information from multiple sensors and sources to improve the reliability and accuracy of defect detection. By combining data from different modalities, such as visual and non-visual sensors, web inspection systems can overcome limitations associated with individual sensing modalities and achieve more comprehensive defect detection coverage.
7. Connectivity and Integration
Modern web inspection systems are often equipped with connectivity features that enable seamless integration with other manufacturing equipment and enterprise systems. Integration with manufacturing execution systems (MES), supervisory control and data acquisition (SCADA) systems, and enterprise resource planning (ERP) systems facilitates data exchange, workflow automation, and decision support, enhancing the overall efficiency and effectiveness of defect detection operations.
Challenges and Solutions of Automated Defect Detection in Web Inspection Systems
1. Variability in Defect Types and Characteristics
- Challenge: Different printing processes and materials can lead to a wide range of defect types and characteristics, making it challenging to develop universal detection algorithms.
- Solution: Employ machine learning and artificial intelligence techniques to train algorithms on a diverse dataset of defects, enabling them to recognize various defect types and adapt to new ones.
2. High-Speed Inspection Requirements
- Challenge: Web printing processes often operate at high speeds, requiring defect detection algorithms to process images quickly and accurately.
- Solution: Utilize parallel processing techniques and hardware acceleration (e.g., GPU computing) to enhance the speed of defect detection algorithms without compromising accuracy.
3. Complex Backgrounds and Patterns
- Challenge: The presence of complex backgrounds and patterns in printed materials can interfere with defect detection, leading to false positives or missed defects.
- Solution: Implement advanced image processing techniques, such as background subtraction, texture analysis, and pattern recognition, to distinguish defects from background noise and patterns.
4. Variations in Lighting and Contrast
- Challenge: Inconsistent lighting conditions and variations in contrast across the web can affect the visibility of defects, making them harder to detect.
- Solution: Employ adaptive imaging techniques, such as dynamic lighting control and contrast enhancement algorithms, to adjust to changing conditions and improve defect visibility.
5. Large Data Volumes and Storage
- Challenge: Web inspection systems generate vast amounts of image data, posing challenges for data storage, management, and processing.
- Solution: Implement efficient data compression techniques, selective data storage strategies, and cloud-based storage solutions to handle large volumes of image data effectively and minimize storage costs.
6. Defect Classification and Prioritization
- Challenge: Not all detected defects are equally critical, and prioritizing them based on severity and impact is essential for efficient quality control.
- Solution: Develop classification algorithms that not only detect defects but also classify them according to their severity and impact on print quality, enabling prioritized inspection and corrective actions.
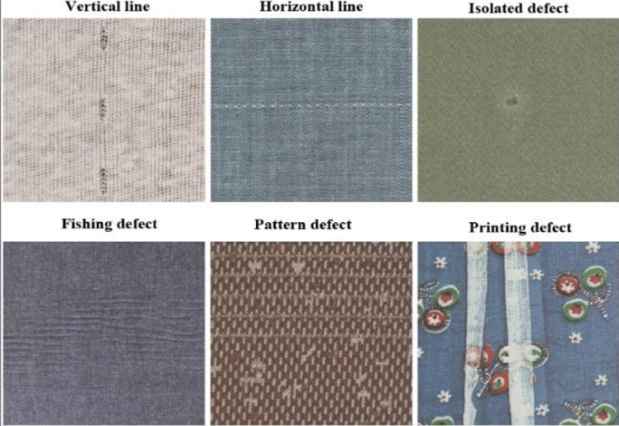
7. Integration with Production Workflow
- Challenge: Seamless integration of web inspection systems into existing production workflows and control systems is crucial for real-time defect detection and process optimization.
- Solution: Utilize standardized communication protocols (e.g., OPC-UA), data exchange formats (e.g., XML, JSON), and interoperability standards to facilitate integration with production equipment and control systems.
Conclusion
Automated defect detection represents a transformative leap in quality control within manufacturing industries reliant on continuous materials, empowering manufacturers to deliver products of unparalleled quality and reliability. As the industry continues to embrace automation and digitalization, automated defect detection will play an increasingly vital role in ensuring excellence across all facets of manufacturing.